U-Net GAN Explained
Par un écrivain mystérieux
Last updated 01 juin 2024

In contrast to typical GANs, a U-Net GAN uses a segmentation network as the discriminator. This segmentation network predicts two classes: real and fake. In doing so, the discriminator gives the generator region-specific feedback. This discriminator design also enables a CutMix-based consistency regularization on the two-dimensional output of the U-Net GAN discriminator, which further improves image synthesis quality.
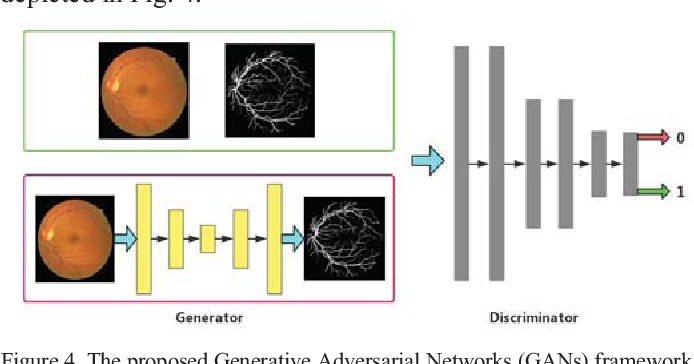
U-GAN: Generative Adversarial Networks with U-Net for Retinal
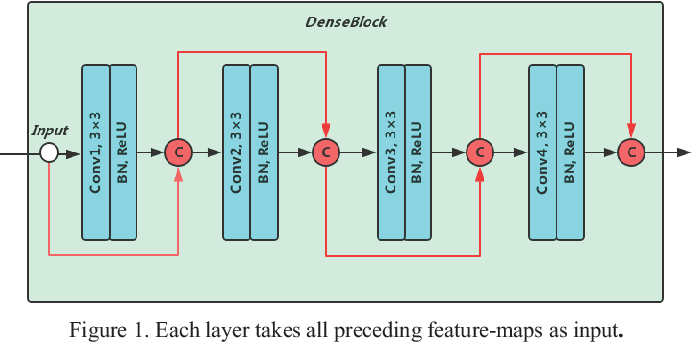
U-GAN: Generative Adversarial Networks with U-Net for Retinal
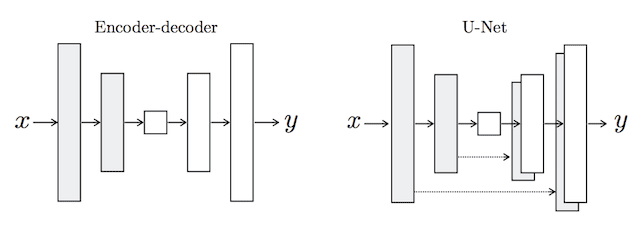
A Gentle Introduction to Pix2Pix Generative Adversarial Network
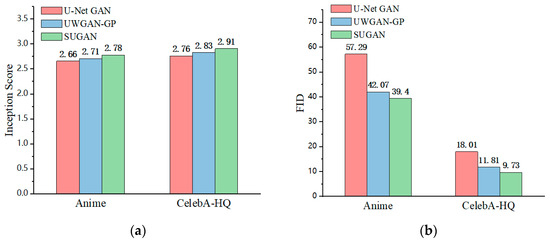
Sensors, Free Full-Text
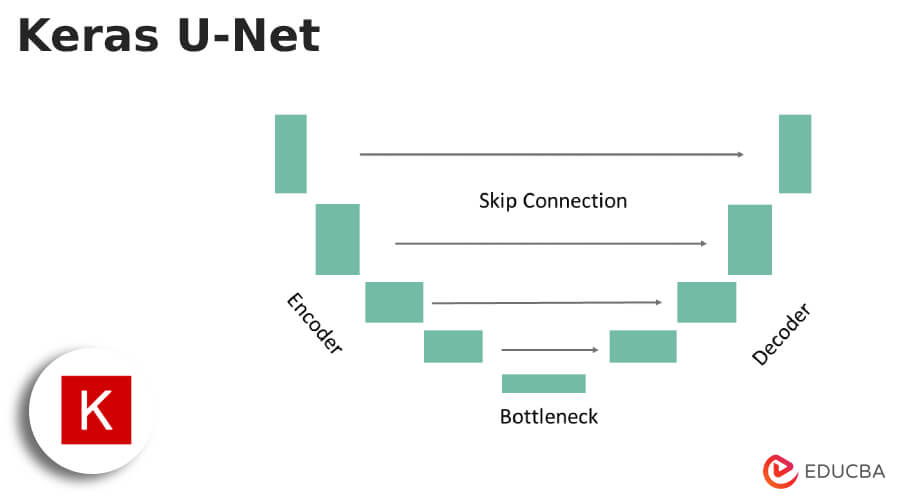
Keras U-Net Image Segmentation with U-Net Architecture
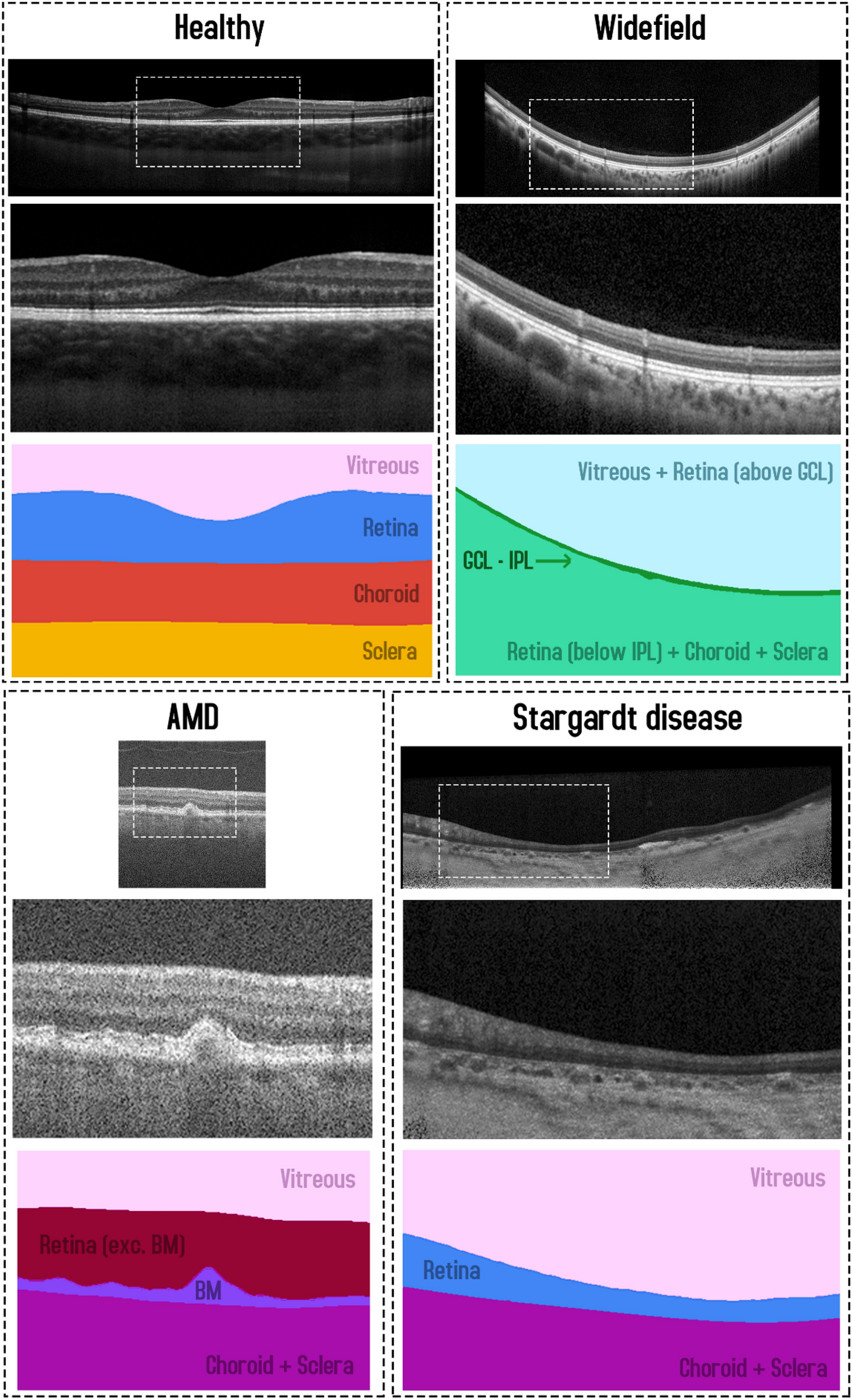
A comparison of deep learning U-Net architectures for posterior

Generating synthesized computed tomography from CBCT using a
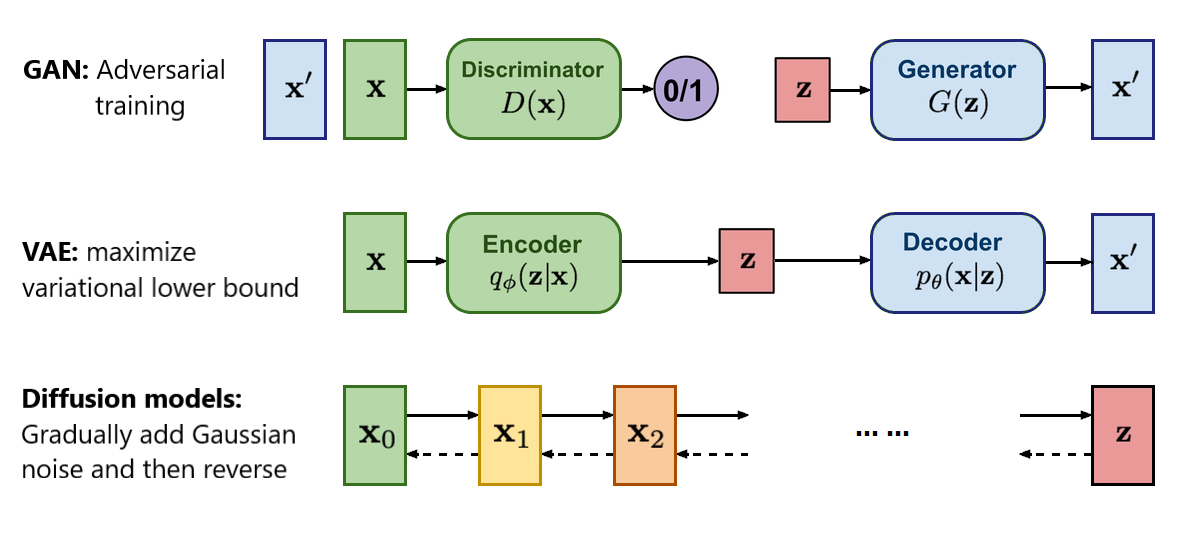
Diffusion Models vs. GANs vs. VAEs: Comparison of Deep Generative
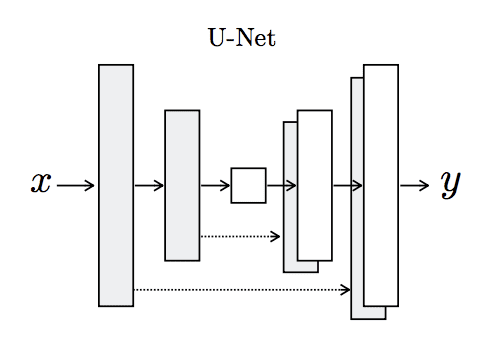
How to Implement Pix2Pix GAN Models From Scratch With Keras
Recommandé pour vous
- GAN Generate Your Own Dataset using Generative Adversarial Networks14 Jul 2023
- The Generator, Machine Learning14 Jul 2023
- Gan 12 UI Free Play14 Jul 2023
- Gan Prévoyance14 Jul 2023
- Malaise social chez Gan Assurances14 Jul 2023
- Gan (Pyrénées-Atlantiques) — Wikipédia14 Jul 2023
- GAN et Machine Learning : Mise en pratique avec des visages fictifs14 Jul 2023
- From GAN to WGAN14 Jul 2023
- Gan - Bastides 6414 Jul 2023
- The proposed Residual Generative Adversarial Network (R-GAN). The14 Jul 2023
Tu pourrais aussi aimer
- Filtre charbon, Hotpoint-Ariston hotte - 190 mm14 Jul 2023
- Comment démonter vitre, coque et moteur d'un rétroviseur de BMW E9014 Jul 2023
- FiiO M15S 5 Inch Bluetooth Portable Music Player — HiFiGo14 Jul 2023
- StarTech.com 6ft 2m Mini DisplayPort to HDMI Cable - 4K 30Hz Mini DP to HDMI Adapter Cable - mDP 1.214 Jul 2023
- dessin-harry-potter-feutres-alcool-les-ateliers-creatifs-villers(2).jpg – Les Ateliers Créatifs14 Jul 2023
- Kojie San - Savons éclaircissants (2 pcs) - 130gr (new packaging) – Ethni Beauty Market14 Jul 2023
- Recharge Exatime 21 - Journalier millésimé Annuel 2024 - Papeterie Michel14 Jul 2023
- Marche Pieds Toyota Yaris Cross 2022 Aujourd Hui Aluminium Art Noir14 Jul 2023
- La Garde du Roi lion : La Grande Bataille - Téléfilm Disney14 Jul 2023
- Gourde Filtrante - Filtre Eau Portable bøyelig Extrieur, Bouteille d'eau filtrante de sport pour camping, randonne, Purificateur D'eau De Survie D14 Jul 2023